(Originally published by Stanford Human-Centered Artificial Intelligence on November 7, 2023)
As a new HAI associate director, the legal scholar sees great potential for AI — but it won’t happen overnight.
Asked to grade the legal profession on how well it’s using AI, Julian Nyarko, an associate professor at Stanford Law School, paused. After some thought, he replied, “B-minus.”
Not bad, but room for improvement.
Nyarko, who recently assumed a role as associate director at the Stanford Institute for Human-Centered AI, studies the wide variety of ways in which AI can be applied in the legal world, from its potential in contract design to issues of algorithmic fairness in the criminal justice system. In conversation with HAI, he describes what the law is getting right — what it’s not — as AI becomes increasingly integral to the profession.
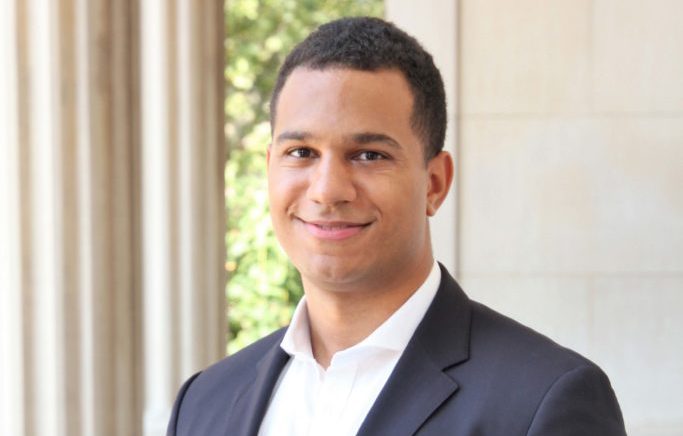
Okay, B-minus. If you had to elaborate…?
For a while, there has been a lot of interest in AI, especially from the commercial side. Big law firms are looking at how to do automated contract review, for instance. And there are a couple of good startups in the works. But I don’t think the ideas or potential have been fully embraced.
That has to do, in part, with the fact that the legal profession is generally somewhat conservative. There are also concerns over questions of liability, which we see playing out, for instance, in the autonomous vehicle sector. Even if autonomous vehicles are safer overall than humans, most people get really angry when they make a mistake that looks like the type of mistake that a human driver would never make. People care more about how this technology operates in the moment, and not so much about aggregate statistics of its performance.
The same holds for law. If you use AI tools that are, on average, better than humans at something like identifying particular risks in a contract, but the algorithm occasionally makes mistakes that a human would never make, then both lawyers and judges get very unhappy about that.
That’s the commercial side. On the government side, particularly in criminal justice, we see all sorts of algorithmic decision rules, and we’ve had these for a while. Even sentencing guidelines can be thought of a form of algorithmic decision making. The challenge here is that there isn’t much expertise within the government, and resources are limited, so the governmental actors increasingly need to rely on collaboration to build their tools. To do that, they turn to academia. To be sure, this is not necessarily a problem. But academia itself can be slow and many of us have limited capacity, so many of the potential gains of AI cannot be realized quickly.
Where are you focusing your efforts right now?
I usually describe my work as falling into three buckets.
The first bucket is how AI has the potential to make law more effective. Take the world of legal doctrine. In law, we often operationalize legal rules by developing certain tests that sound like they can be empirically verified. In trademarks, for instance, we have this concept of a “genericide.” In essence, a genericide is a protect mark that becomes so ubiquitous that it can lose its protection. Take, for instance, Kleenex. Everyone knows that Kleenex is a type of tissue. But what does someone mean who says: “This movie was so sad, can you grab me a Kleenex?” Do they want the particular Kleenex brand, or are they just using the word Kleenex as a stand-in for generic tissues, irrespective of the brand? If it is the latter, and that behavior becomes widespread among consumers, Kleenex can actually lose its trademark protection. This happened, for instance, with escalator, which was once a particular brand of electrical staircase.
We see that a key legal test for genericide has to do with understanding how consumers use the word Kleenex. What happens in their minds? This sounds like something that can be objectively verified, but what often happens in courts is we run surveys that are not well-designed or we invite experts who simply voice an opinion — one that happens to be especially favorable for their client.
In fact, we have a wealth of data that tells us how people use these words. AI tools can help us get a handle on this. For instance, you can ask a language model to predict the probability of the word that comes next in this sentence: “This movie is so sad that I reach to grab a [blank].” Knowing that can give us a sense of whether there is a difference in how people use Kleenex and tissue, which in turn offers a window into consumers’ minds. This is a more rigorous way, through language, to infuse legal tests with more objectivity.
And your other buckets?
The second bucket I think of as augmenting AI for legal applications. How do we tweak models for legal applications? How do we establish benchmarks to even know that they work?
For example, law often involves long documents, but the longer the document, the more expensive it is for a language model to review it. The way many of these models work is they try to make a determination based on all of the text that is provided as an input. The more these models have to keep in their mind, so to speak, the larger the cost of adding information.
One thing I’m working on to simplify this is devising ways to split long documents into chunks, quickly identify only the relevant parts, and use those in the language model. In effect, this allows you to analyze a long document at only a fraction of the cost.
The third bucket is about fairness. To give an example: Many of our anti-discrimination laws were developed in the context of human decision-making and focus on discriminatory intent. But with an algorithm, the idea of intent doesn’t make sense. It has no intent toward one group or another. So how do we think about questions of fairness when it comes to AI and the law? Is the law ready for the emerging algorithmic age, and how are algorithms spreading through the legal landscape?
Do you see places where AI is being misapplied, or where people have misguided expectations about its value?
The Holy Grail for many people who work on AI in the law is something like litigation outcome prediction. They want a tool that can take a set of facts and tell you, for instance, whether to sue or what the odds of success are. This is a task that involves a lot of what we often refer to as “legal reasoning.” And I think we’re still far away from AI being used as a tool for more complex legal reasoning. There are lots of interesting demonstrations where GPT passes the Bar, for example, but these are largely driven by performance on multiple choice. The model is also very good at classifying and defining the relevant rules. But when you describe a more complex set of facts to these models, they have a much harder time deciding whether the relevant rules apply. They can tell you what a penalty clause is in a contract, but when asked whether a penalty applies under given circumstances, the answers we get aren’t good.
For the legal sector right now, this means we need to think carefully about how and where to deploy AI for specific tasks. Information extraction from large texts is one promising area, so lawyers don’t have to read a whole document.
Do you see specific areas where you think people should slow down because the tools or the law aren’t yet ready?
Going back to a previous point, I think there is still a lack of clarity around how many legal constraints that make sense for human decision making should apply to AI-based decision making. Take, for instance, credit risk. There are many FinTech lenders who are trying to use AI to better estimate an applicant’s credit risk. These FinTech lenders operate very differently from traditional banks, and we now see that many rules from traditional anti-discrimination laws can lead to unintended outcomes in that context. For instance, a key number that courts care about when they assess discriminatory lending practices is the decision rate for white applicants and the minority group. For instance, if 9% of white applicants get a loan, while only 4% of Black applicants get a loan, that is viewed as potentially problematic.
Now FinTech lenders have a lot of control over the applicants they get. Imagine they want to do community outreach in under-resourced, Black communities. What that will do is drive up the number of Black applicants who will get credit, which is good. But at the same time, it might also drive down the share of Black applicants who qualify for a loan, simply because there are many high-risk lenders in that community. In effect, anti-discrimination laws might discourage FinTech lenders from outreach, pushing them to focus on communities that are well-resourced and predominantly white. These types of issues have always been potentially problematic, but in an age of AI-driven decision making, they become much more glaring. It becomes obvious that the law is not ready.
I don’t know if that means we have to slow down, exactly, but we do need to get legal clarity around how these ideas interact.
You’re now a leader at Stanford HAI. What does the term “human-centered AI” mean to you?
What’s most important when I think about AI — and this is consistent with the mission of HAI — is that it ultimately is a tool that should be used to improve what I call welfare in the world. That’s the goal: promote welfare, promote happiness and health among people, and so on.
The alternative is to focus on simply developing the best tool possible without worrying about implementation or how it will be used. But with this approach you might optimize toward some goal that ultimately makes the world a worse place.
Focusing on welfare may mean that we have to make algorithms that don’t perform as well as they could, but welfare needs to be the target of our efforts. We optimize based on that. People often say: “Well, my job is to optimize the algorithm and it’s someone else’s job to think about implications.” But that can yield poor results. In my view, for an AI tool to be truly useful, it needs to be constructed from the ground up with human welfare in mind.
Stanford HAI’s mission is to advance AI research, education, policy and practice to improve the human condition. Learn more.
This post was originally published on this site be sure to check out more of their content.