Does A.I. Lead Police to Ignore Contradictory Evidence?
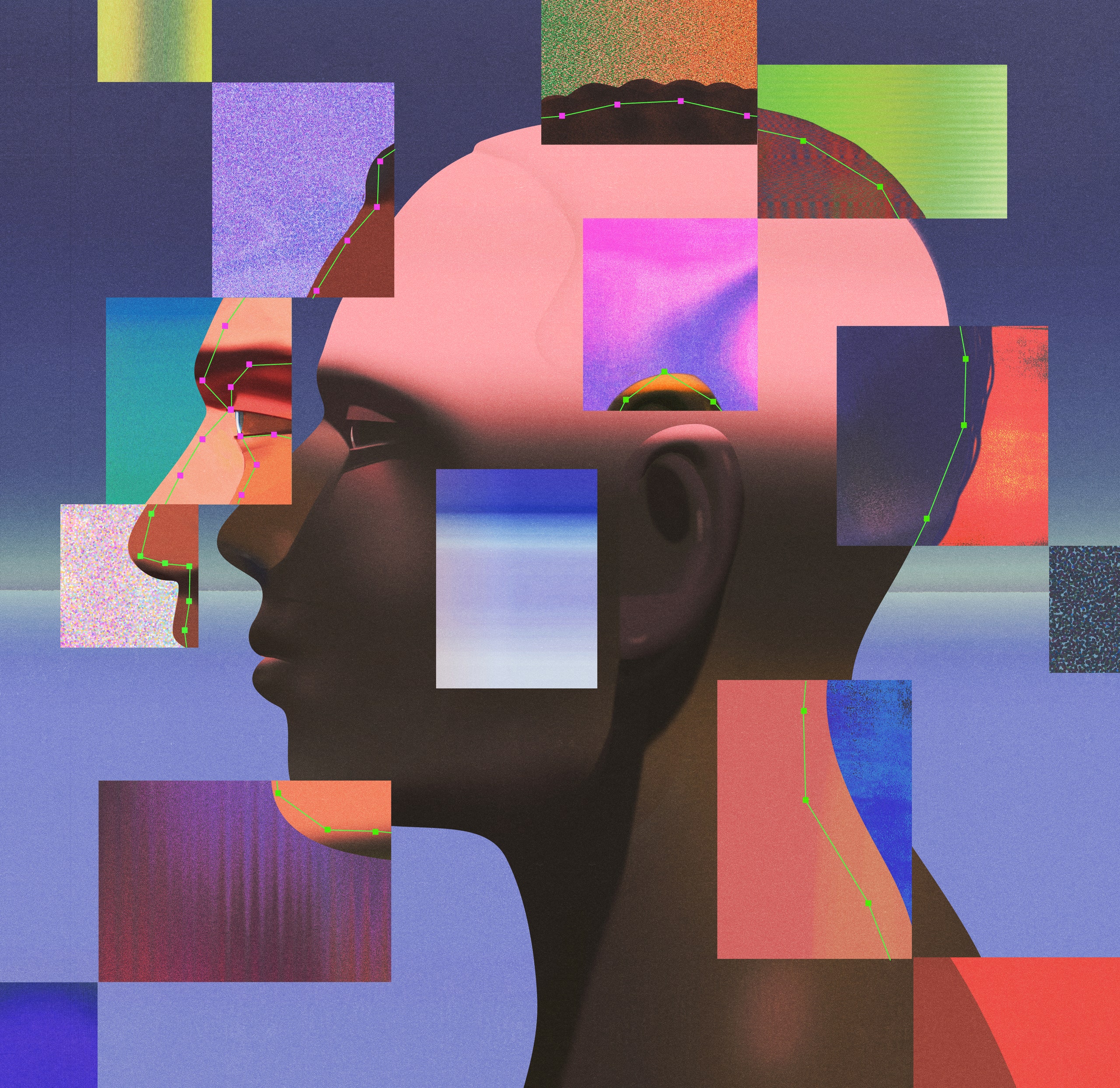
On March 26, 2022, at around 8:20 a.m., a man in light-blue Nike sweatpants boarded a bus near a shopping plaza in Timonium, outside Baltimore. After the bus driver ordered him to observe a rule requiring passengers to wear face masks, he approached the fare box and began arguing with her. “I hit bitches,” he said, leaning over a plastic shield that the driver was sitting behind. When she pulled out her iPhone to call the police, he reached around the shield, snatched the device, and raced off. The bus driver followed the man outside, where he punched her in the face repeatedly. He then stood by the curb, laughing, as his victim wiped blood from her nose.
By the time police officers canvassed the area, the assailant had fled, but the incident had been captured on surveillance cameras. Officers with the Maryland Transit Administration Police extracted still images from the footage and created a Be on the Lookout bulletin, which was disseminated to law-enforcement agencies. It included several pictures of the alleged perpetrator: a slender Black man whose face was partially obscured by a baseball cap and a hoodie. The bulletin was also sent to the state’s attorney’s office of nearby Harford County, and an analyst there decided to run a facial-recognition search. She fed a still image into software that used algorithms to identify faces that had similar characteristics in a vast database of pictures. This “probe photograph” generated a list of potential matches. (Researchers have identified roughly eighty “nodal points” that convey the distinct geometry of a human face.) The match that stood out to the analyst was Alonzo Cornelius Sawyer, a Maryland resident in his mid-fifties.
On March 28th, Sawyer became a person of interest in the case, and his name was forwarded to the M.T.A.’s criminal-investigation unit, where detectives combed through police databases for information about him. They discovered that he had recently been on probation for a string of traffic violations. In three days, he was scheduled to answer a summons by appearing at a courthouse in Harford County, after being stopped for allegedly driving without a license. Sawyer showed up at the hearing in good spirits, laughing with a guard at the entrance. On his way out, after he’d learned that his case had been postponed, a U.S. deputy marshal grabbed him from behind, slammed him against a wall, and asked him if he was Alonzo Sawyer. “Yeah,” Sawyer said. The marshal told him that he had a warrant for his arrest. “Tell me what it’s about,” Sawyer pleaded. The marshal told him that he’d find out soon enough.
Sawyer was handcuffed and taken to the M.T.A.’s police headquarters, in Baltimore, where two officers interrogated him about where he’d been on March 26th. Sawyer said that he and his wife, who were about to move into a new apartment, had been staying at the house of his sister-in-law, who lived in Abingdon, a suburb forty minutes northeast of Baltimore, in Harford County. But he could not remember if he’d spent the entire day there. When was the last time he’d been in Baltimore County? Sawyer said that he couldn’t recall, but insisted that he didn’t ride M.T.A. buses and hadn’t been involved in a confrontation that day. The officers then showed him the Be on the Lookout bulletin, and one of them asked, “Then who was this?” Sawyer stared at the photographs and said, “I don’t know—who is it?” Like him, the man had a thin face and a goatee. But he looked no older than thirty-five, Sawyer thought—young enough to be his son. And although their skin color was similar, the color of their clothing was not. Pointing at the assailant’s sweatpants, he said, “I don’t wear light blue—I don’t even own anything in that color.”
The officers weren’t persuaded. Sawyer was transported to the Baltimore County Detention Center and charged with two counts of second-degree assault and multiple charges related to the theft of the phone. He was denied bail, owing to the viciousness of the crime, which carried a potential twenty-five-year sentence.
In 2016, the Center on Privacy and Technology, at Georgetown University Law Center, published a report, “The Perpetual Line-Up,” which estimated that the faces of a hundred and seventeen million Americans were in facial-recognition databases that state and local law-enforcement agencies could access. Many of these images came from government sources—driver’s-license photographs, mug shots, and the like. Other pictures came from sources such as surveillance cameras and social media.
In the years since the report’s publication, the technology has only grown more ubiquitous, not least because selling it is a lucrative business, and A.I. companies have successfully persuaded law-enforcement agencies to become customers. A 2021 investigation by BuzzFeed News found that employees at nearly two thousand public agencies had used or tested software developed by Clearview AI, a facial-recognition firm with a database containing billions of images that have been scraped off the Internet. The company has marketed its services to the police by promising that its software is “100% accurate across all demographic groups.”
Proponents view facial-recognition technology as an invaluable tool that can help make policing more efficient and insure that criminals are held accountable. The technology’s reputation got a boost after it helped investigators identify numerous rioters who stormed the U.S. Capitol on January 6, 2021. In “Your Face Belongs to Us,” a new book that traces the history of facial-recognition technology, Kashmir Hill, a reporter at the Times, describes how, in 2019, a Department of Homeland Security agent investigating a child-sex-abuse case e-mailed a suspect’s photograph to colleagues, one of whom ran the image through Clearview AI’s platform. The agent received back an Instagram photograph of a muscular man and a muscular woman posing at a bodybuilding expo in Las Vegas. In the background of the image was someone who resembled the suspect; he was standing behind a table at the booth of a dietary-supplement company. The agent called the company, which was based in Florida. The man, identified as Andres Rafael Viola, was arrested, and in his subsequent trial federal authorities presented enough other evidence, such as images obtained from his electronic devices, to secure a conviction. Viola was sentenced to thirty-five years in prison.
It’s not hard to imagine why law-enforcement officials might desire a tool capable of such feats. Critics, however, fear that the police could use automated face recognition for more objectionable purposes, such as monitoring the activities of peaceful protesters and impinging on citizens’ privacy. And questions remain about how reliable the tool is. Like all machine-learning systems, facial-recognition software makes predictions by discerning patterns in large volumes of data. This analysis is often done using artificial neural networks, which mimic the function of the human brain. The technology is trained with photographs of faces, just as ChatGPT is trained with text, and builds a statistical model that can assign a confidence score to indicate how similar two images are. But even a confidence score of ninety-nine per cent isn’t a guaranteed match. The companies that market such technology acknowledge that the score reflects an “algorithmic best guess”—one whose accuracy may vary depending on the quality of the probe photograph, which can be compromised by factors such as lighting and camera angle. Moreover, if the data set used to train the algorithm is imbalanced—more male faces than female ones, or more white faces than Black ones—the model may perform worse for some demographic groups. Jonathan Frankle, a neural-networks specialist who has researched facial-recognition technology, told me, “As with all things in machine learning, you’re only as good as your data. If my training data heavily represents a certain group, my model will likely be more reliable at assessing members of that group, because that’s what it saw.”
In January of 2020, a resident of Michigan named Robert Williams was arrested in front of his wife and children for allegedly stealing watches from a store in Detroit, after a facial-recognition search, based on a photograph extracted from surveillance footage, identified him as a person of interest. Williams did not commit the crime, as the police realized when he held up a picture of the shoplifter next to his face: among other things, Williams was larger than the thief and had a broader face. His ordeal, later recounted in the Times, was the first publicly known example of a case in which facial-recognition technology played a prominent role in a false arrest. Five similar cases have since been documented. In all of them, the suspect mistakenly brought into custody has been Black, raising concerns that the algorithms less accurately distinguish the faces of people with darker skin, reinforcing racial disparities in the criminal-justice system. In 2019, the National Institute of Standards and Technology, a federal agency, published a study revealing that many facial-recognition systems falsely identified Black and Asian faces between ten and a hundred times more frequently than Caucasian ones. Errors were also more common for women and elderly people.
Advocates of facial-recognition technology acknowledge that the quality of the algorithms varies greatly, but they contend that the best ones do not have such demographic imbalances. They also note that, among the millions of searches that have been conducted by police, only a few have been proved to lead to wrongful arrests. But how many people have been erroneously identified without the mistake being recognized? Nobody can say, in part because the technology is poorly regulated and the police’s use of it is often not shared with either the public or the accused. Last fall, a man named Randal Quran Reid was arrested for two acts of credit-card fraud in Louisiana that he did not commit. The warrant didn’t mention that a facial-recognition search had made him a suspect. Reid discovered this fact only after his lawyer heard an officer refer to him as a “positive match” for the thief. Reid was in jail for six days and his family spent thousands of dollars in legal fees before learning about the misidentification, which had resulted from a search done by a police department under contract with Clearview AI. So much for being “100% accurate.”
Law-enforcement officials argue that they aren’t obligated to disclose such information because, in theory at least, facial-recognition searches are being used only to generate leads for a fuller investigation, and do not alone serve as probable cause for making an arrest. Yet, in a striking number of the wrongful arrests that have been documented, the searches represented virtually the entire investigation. No other evidence seemed to link Randal Reid, who lives in Georgia, to the thefts in Louisiana, a state he had never even visited. No investigator from the Detroit police checked the location data on Robert Williams’s phone to verify whether he had been in the store on the day that he allegedly robbed it. The police did consult a security contractor, who reviewed surveillance video of the shoplifting incident and then chose Williams from a photo lineup of six people. But the security contractor had not been in the store when the incident occurred and had never seen Williams in person.
The Maryland Transit Administration Police did try to find other evidence linking Alonzo Sawyer to the assault on the bus driver. On the day he was arrested, two M.T.A. officers, Ashleigh Tarrant and Ryan Naglieri, drove to Abingdon to vet his alibi. They visited the home of Donna Ogala—the older sister of Sawyer’s wife, Carronne Jones—and asked for consent to search the premises. Ogala invited them inside. In the living room, they found piles of blankets and clothing strewn around a gray sectional couch, which Sawyer and his wife had been using as a makeshift bed. But, as Detective Tarrant later noted in an account of the visit, he and Naglieri did not find anything incriminating. “I could not locate any clothing that the suspect had on in the Be on the Lookout bulletin,” he wrote.
Before leaving, the officers questioned Ogala, a retired public-school teacher, about the morning that the bus assault took place. At 7:45 a.m., she told them, she went downstairs to the kitchen to take her diabetes medication, as she did every morning, and saw Sawyer and her sister asleep on the couch. At around nine-thirty—well after the assault in Timonium had happened—she went out to buy something at Walmart. Sawyer and her sister still hadn’t gotten up.
Investigators also reached out to LaDonna Wilson, the bus driver. The day after searching Ogala’s home, the police showed Wilson a six-person photo lineup, to see if she would identify Sawyer. The third person in the lineup, a copy of which I obtained through a public-records request, was Sawyer—his head shaved, his brow creased, a trace of gray in his neatly trimmed goatee. Beneath each photograph, you could check “yes” or “no.” After examining the picture, Wilson checked “no.” Then she wrote, “Not the person who assaulted me.” (Wilson declined to be interviewed for this article.)
As Sawyer sat in jail, none of this information was relayed to him. One thing he was told is that the police had found someone who’d confirmed the facial-recognition match and had identified him as the person in the Be on the Lookout bulletin. The confirmation came from Arron Daugherty—his probation officer. On March 29th, two days before Sawyer was brought into custody, a copy of the bulletin was e-mailed to Daugherty. He responded that the assailant looked like Sawyer, though he was not certain that it was him. He asked to see the surveillance video of the crime. Later that day, Detective Tarrant visited Daugherty at his office, in Hagerstown, and showed him the video. After watching it, Daugherty signed a document attesting that he’d identified Sawyer as the suspect.
Daugherty’s confirmation may explain why the police dismissed Sawyer’s claim that he had been misidentified. But how qualified was Daugherty to settle the matter? According to Sawyer, they had met in person only twice. On both occasions, Sawyer had worn a face mask, because of pandemic restrictions. If the brevity of these interactions failed to give investigators pause, it may well be because the results of the facial-recognition search had already convinced them of Sawyer’s guilt. A.I. tools have benefitted from what researchers call “automation bias”: the inclination of people using computer technology to uncritically accept what machines tell them, especially when they perform functions that are inscrutable to them. Clare Garvie, an attorney with the National Association of Criminal Defense Lawyers, studies the intersection of technology and due-process rights, and she told me, “Facial-recognition technology has a kind of mystery to it—we don’t really know how it operates, but we know that people much smarter than us created it. There’s a tendency to place trust in a system that we don’t fully understand.”
In a report published last year, Garvie argued that the technology’s presumed infallibility risked obscuring the fact that, in the context of criminal investigations, facial recognition is a tool that depends heavily on subjective human judgment. The typical search generates not just a single face but, rather, a “candidate list” of dozens, sometimes hundreds, of potential matches. Most of the returns, in other words, are false positives. The task of reviewing the list and deciding which, if any, candidate might be a correct match falls not to an algorithm but to a person—a law-enforcement representative whose qualifications in this area might be limited. A report published in September by the Government Accountability Office found that seven law-enforcement agencies in the Department of Homeland Security and the Department of Justice initially allowed staff to use facial-recognition technology without any training. The Federal Bureau of Investigation did require some agents to take a twenty-four-hour course, but the G.A.O. revealed that only ten of the hundred and ninety-six staff members with access to the technology had completed it.
If comparing and identifying unfamiliar faces were tasks that human beings could easily master, the lack of training might not be cause for concern. But, in one study in which participants were asked to identify someone from a photographic pool of suspects, the error rate was as high as thirty per cent. And the study used mainly high-quality images of people in straightforward poses—a luxury that law-enforcement agents examining images extracted from grainy surveillance video usually don’t have. Studies using low-quality images have resulted in even higher error rates. You might assume that professionals with experience performing forensic face examinations would be less likely to misidentify someone, but this isn’t the case. A study comparing passport officials with college students found that the officers performed as poorly as the students.
Another factor that can increase the risk of mistakes is the “cross-race effect”: the tendency of humans to have difficulty recognizing the faces of people of a different race or ethnicity. A review of fourteen experimental studies found that about eighty per cent of both white and Black subjects were better at distinguishing faces of their own race. “If the face recognition analyst is white and the subject of the search is Black, or vice versa, the reliability of the search may be particularly suspect,” Garvie’s report noted.
Detectives who receive investigative leads generated by facial-recognition technology may not be aware of any of these pitfalls, and may assume that an A.I. search has found an exact match rather than, say, a similar-looking person whose picture, taken from social media, does not reflect the person’s current appearance. The photograph of Robert Williams that led to his arrest for robbing the Detroit store came from an old driver’s license. The analyst at the Michigan State Police who conducted the search didn’t bother to check whether the license had expired—it had. Nor did she appear to consider why more recent pictures of Williams, which were also in the database, didn’t turn up as candidates. The dated picture of Williams was only the ninth most likely match for the probe photograph, which was obtained from surveillance video of the incident. But the analyst who ran the search did a morphological assessment of Williams’s face, including the shape of his nostrils, and found that his was the most similar to the suspect’s. Two other algorithms were then run. In one of them, which returned two hundred and forty-three results, Williams wasn’t even on the candidate list. In the other—of an F.B.I. database—the probe photograph generated no results at all.
Nathan Freed Wessler, an attorney at the A.C.L.U., is now representing Williams in a lawsuit. He suspects that the reason the F.B.I. database came up empty is that the probe photograph was of extremely poor quality. The fact that Williams didn’t appear in two of the three facial-recognition searches came to light only recently, during the pretrial discovery process, as the A.C.L.U. pressed the Michigan State Police for more detailed information about the results. If not for the litigation, Williams and his family would likely never have learned about the finding.
When the police called Carronne Jones, Sawyer’s wife, to inform her that her husband had been arrested, she rushed to the M.T.A.’s police headquarters, where she met with Detective Tarrant and Lieutenant Kenneth Combs, the officers who’d questioned Sawyer. When they informed her of what he had allegedly done, she was astonished. Her husband didn’t ride buses, she told them, and he didn’t hit women. The officers confidently showed her the Be on the Lookout bulletin and asked, But isn’t this him? After glancing at it, Jones strained not to let her anger show. Her husband was older, she pointed out, with more facial hair and a prominent gap between his teeth that the suspect didn’t have.
The officers then showed her the video of the assault. Jones immediately noticed that the man in the footage was dressed in clothing that Sawyer didn’t own. She also pointed out that the assailant hadn’t ducked his head when he’d got on the bus, as Sawyer, who is six feet six, surely would have done. Jones asked in exasperation, “Why are my husband and I even here?” They looked at her dismissively, she told me. (The M.T.A. did not make Tarrant and Combs available for interviews.)
Like Sawyer, Jones is in her fifties and has a reserved manner, but when something upsets her enough she pushes back fiercely. After speaking with the police, she was livid, but she was also afraid that nobody would believe her or her husband. In desperation, she reached out to a lawyer to get Sawyer’s bail status reconsidered. At a hearing, the lawyer presented a statement from Jones’s sister that she had seen Sawyer sleeping on her couch on the morning of the crime, but a judge refused to change the bail terms.
A few days later, Jones drove to Hagerstown to speak with Arron Daugherty, Sawyer’s probation officer. “How can I help you?” he asked. When she explained who she was, he was clearly surprised. She told him the police had informed her that her husband was facing decades in prison because of Daugherty’s “positive I.D. match.” How could he be certain if he’d never even seen Sawyer without a face mask? Jones told me that Daugherty denied making a definitive identification and noted that he’d told the police he wasn’t sure if the male in the Be on the Lookout bulletin was her husband. (Daugherty declined to answer questions for this article.) If that was the case, she demanded, why had he signed an official document confirming that Sawyer was the perpetrator? According to Jones, Daugherty said that when the police officers had shown him the surveillance footage of the bus incident they had suggested that the assailant was Sawyer. Before leaving, Jones showed Daugherty some photos of Sawyer that she’d brought along. “This is my husband,” she said. She also announced that if the state went ahead and prosecuted him she would make sure that someone was held accountable.
The next day, Daugherty sent an e-mail to Detective Tarrant, who had shown him the video of the assault on the bus. “Mr. Sawyer’s wife came to the office yesterday afternoon to see me,” it stated. “She provided me with additional photos of Mr. Sawyer. I reviewed the photos . . . and have doubts that the person in the BOLO”—the Be on the Lookout bulletin—“is Mr. Sawyer.”
Daugherty’s e-mail was sent to the Maryland Transit Administration Police on April 5th. A few days later, Alonzo Sawyer was released from jail.
Scott Shellenberger, the state’s attorney in Baltimore County, told me that the probe photograph that was used in Sawyer’s case had been extracted from “grainy surveillance video.” Once doubts arose about the identity of the subject, Shellenberger added, the case “got dismissed pretty quickly.” But the process did not seem quick to Sawyer, whom I met not long ago at a church in Harford County. He was wearing a Baltimore Orioles cap and a short-sleeved black shirt adorned with the logo of his employer, Bemo Solutions, a lighting-installation company. Sawyer had just finished working and greeted me with a weary smile. Then he escorted me through the doors of the church and into a small room appointed with two gray armchairs and a navy-blue couch. His wife was on the sofa. After folding his long frame into one of the chairs, he recounted his ordeal.
He told me that when the deputy marshal had thrown him against a wall and told him a warrant had been issued for his arrest, his first thought was “Something crazy’s goin’ on.” His bewilderment deepened after he was brought into custody and asked to describe his whereabouts during the week of the bus incident. As the officers pressed him for details, he froze, both because he was shaken and because he didn’t remember the events of that day with confidence, and was afraid of making a false statement. Later, after he was denied bail, it hit him that he might be spending the rest of his life in prison for something that he didn’t do. This, he told me, was the ultimate nightmare of every Black man he knew: “All I thought is ‘Black man, gone—lost in the system.’ ”
Sawyer motioned toward Jones. If she hadn’t intervened, he said, “I’d-a ate that.” By this, he meant that he would have pleaded guilty to the charges and accepted a lower sentence, in exchange for avoiding the risk of a trial. “If it hadn’t been for her, I would have taken a plea,” he said. “I wouldn’t have had a choice, ’cause twenty-five years is a long time.”
This is, in fact, what the vast majority of people facing criminal charges do. Just two per cent of criminal cases go to trial. The rest end in plea-bargain deals, in which defendants receive lower sentences and forgo the opportunity to contest the charges. “In how many of those cases was somebody taking a plea for something they didn’t do?” Clare Garvie asked me. “We quite simply have no transparency into the use of face recognition and how many times it’s made mistakes.”
The lack of transparency can be traced to the fact that, as with other forms of artificial intelligence, the use of facial-recognition software has outpaced a willingness to regulate it. Charles Sydnor III, a state senator in Maryland, told me that many lawmakers are loath to place constraints on technology they do not understand but have been assured can deter crime. Sydnor first grew concerned about face recognition in 2016, when he came across a copy of “The Perpetual Line-Up,” the report published by the Center on Privacy and Technology. “No state has passed a law comprehensively regulating police face recognition,” the report noted, even though at least a quarter of local and state police departments had access to the technology. The next year, Sydnor proposed a moratorium on facial-recognition searches until its potential harms were better understood. The bill got no support, he told me. Eventually, he decided to push for legislation that would permit law enforcement to use facial recognition but also establish guidelines to prevent abuse, such as requiring the police to corroborate a match with independent evidence before making an arrest. (The Maryland Senate approved the legislation last year, but the bill died after the House added an unpopular amendment that would have lessened penalties for people caught failing to stop at the flashing lights of a school bus.)
If police departments took such regulation seriously, it would be a meaningful step. But Nathan Wessler, of the A.C.L.U., told me that the police have often interpreted such guidelines to mean merely “face recognition plus a photo lineup.” If witnesses are told something suggestive—or shown a lineup in which the only person who resembles the culprit is the algorithm’s choice—they will likely confirm a false match. The A.C.L.U. advocates barring facial-recognition technology. “We should at least press Pause until lawmakers can sort this out,” Wessler said. Bans have been enacted in more than twenty cities; many of them are extremely liberal (and predominantly white) places, such as Northampton, Massachusetts, and Madison, Wisconsin. And Vermont recently prohibited the technology’s use in all criminal investigations except in cases involving the sexual exploitation of children.
At the federal level, however, efforts to limit the use of facial-recognition technology have stalled, even though both Democrats and Republicans have voiced concerns about its effects on civil rights and privacy. At a congressional hearing in 2019, Jim Jordan, the hard-right representative from Ohio, said, “It doesn’t matter what side of the political spectrum you’re on—this should concern us all.” But Congress has still not passed any legislation. Andrew Guthrie Ferguson, a professor at the American University Washington College of Law who testified at that hearing, told me that opponents of the technology had pushed for a moratorium even though there clearly wasn’t sufficient support. Tech companies cynically welcomed this strategy, he added, seeing it as a way to prevent any regulation from passing. “There have been some thoughtful compromise bills before Congress, but they haven’t gone forward because of this all-or-nothing approach,” Ferguson said.
In parts of the country where concerns about crime outweigh concerns about prosecutorial abuses, advocates are trying to limit the technology’s use to certain felonies and to regulate it stringently. In Montana, a bipartisan group of legislators passed a law that restricts facial-recognition technology’s use to a designated list of “serious crimes,” such as rape and homicide. (It can also be used to find a missing or deceased person.) To conduct a search, law enforcement needs to have probable cause and a warrant. Searches through third-party venders such as Clearview AI—which has collected photographs from public social-media accounts without users’ permission—require special approval. To allay concerns about improper surveillance, the Montana police are barred from mounting cameras on public buildings.
Software engineers familiar with how rapidly artificial intelligence is advancing might feel that, as the technology improves and the algorithms grow more accurate, such restrictions will be unnecessary. Jonathan Frankle, the neural-networks specialist, disagrees. “ChatGPT is not being trained to make decisions about people’s life and liberty,” he noted. “The risks here are far greater.” Studies have now confirmed that the danger is especially pronounced for members of certain communities. A review of a facial-recognition program in San Diego, which allowed the police to take pictures of suspected criminals “in the field” and run them against a mug-shot database, found that a person of color was between one and a half and two and a half times more likely to have his face entered into the system by this method. (A suspension was placed on the program in 2020, but it has since expired, leaving legislators at a crossroads.) “Community members who are already marginalized will be targeted,” Tawana Petty, a social-justice organizer in Detroit, told me. In 2016, the Detroit police launched Project Green Light, a crime-control initiative that placed surveillance cameras at gas stations in inner-city neighborhoods. The project was “presented to us as safety,” Petty said. At least three Black people in Detroit have since been wrongfully arrested—including, most recently, a woman named Porcha Woodruff, who was eight months pregnant at the time.
Until Alonzo Sawyer’s face turned up in a search, he had never heard of facial-recognition technology. “I wanna know whose idea it was,” he told me, adding that he didn’t fully understand how a computer made such matches. According to the Maryland Department of Public Safety & Correctional Services, the vender that the state was using at the time of Sawyer’s arrest was a company called DataWorks Plus. It is based in Greenville, South Carolina, and provides services to more than twenty-five hundred public agencies. The company’s Web site states that it “uses the latest facial matching technology to provide accurate, reliable facial candidates with advanced comparison, editing, and morphological analysis tools for investigations.” I reached out to the firm repeatedly to find out more about the process that led to Sawyer’s arrest. I didn’t hear back. I also contacted the Harford County state’s attorney’s office, after learning that an intelligence analyst there named Meghan Ryan had conducted the search in Sawyer’s case. Ryan no longer worked at the agency, I was told. When I asked for information about the search—the probe photograph, the candidate list—I was told that no records were available.
In June, an appellate court ordered the N.Y.P.D. to turn over detailed information about a facial-recognition search that had led a Queens resident named Francisco Arteaga to be charged with robbing a store. The court requested both the source code of the software used and information about its algorithm. Because the technology was “novel and untested,” the court held, denying defendants access to such information risked violating the Brady rule, which requires prosecutors to disclose all potentially exculpatory evidence to suspects facing criminal charges. Among the things a defendant might want to know is whether the photograph that had been used in a search leading to his arrest had been digitally altered. DataWorks Plus notes on its Web site that probe images fed into its software “can be edited using pose correction, light normalization, rotation, cropping.” Some systems enable the police to combine two photographs; others include 3-D-imaging tools for reconstructing features that are difficult to make out.
To this day, little about the facial-recognition search has been disclosed to Sawyer. Not long ago, I met with him again, this time in Washington Park, a low-income housing complex in Baltimore, where he’d grown up. He’d lived there with his grandmother, he told me, on the second floor of a building with yellow siding and a bright-blue entrance door. Back then, the door was metal and had a bullet hole above it, he said. In the building next door, addicts shot up in a stairwell. In another unit, lethal fights with baseball bats broke out. “It was rough,” Sawyer recalled as we strolled around. But it was better than living in North Carolina with his mother, who was in an abusive relationship. Sawyer, who has struggled for years with alcoholism, stopped drinking a year ago; a counsellor had helped him see that liquor was a way to avoid contending with “unhealed trauma.” Alcohol had figured in a number of his traffic offenses, I subsequently learned, including several D.U.I. arrests that had eventually led to the suspension of his driver’s license.
When I described Sawyer’s case to Alice Towler, a cognitive scientist who studies facial recognition in humans, she raised the possibility that the driving infractions could have led whoever conducted the search to land on him as a potential suspect: an analyst who was privy to this information may have assumed that Sawyer had had no choice but to take the bus on the morning of March 26th, and was angry about it. Some facial-recognition systems even display information about prior arrests alongside each photograph in the candidate list. This might seem like a good way to help the person reviewing the results make a more informed decision. But Towler told me that such contextual information can also foster cognitive bias—leading an analyst to select the person whose biography tells the best story. “Circumstantial evidence can leak into the perceptual judgment,” she said.
Sawyer’s prior arrests may have also increased the odds that his face would appear in a database of mug shots. Black people are overrepresented in such databases, in part because they live in communities that are overpoliced. “The more times you engage with the police, the more times you’re picked up, the more tickets you’ve bought to the misidentification lottery,” Clare Garvie said. One way to mitigate this problem would be to restrict searches to databases in which all citizens are equally represented, such as those containing driver’s-license photos. Proposed legislation in Massachusetts would essentially require this. In jurisdictions without such constraints—most of America—the person most likely to be misidentified by facial-recognition technology is not a random white citizen with a clean record and an Instagram account; it’s a person of color with a long rap sheet.
Sawyer said that, as surprised as he’d been about the technology behind his false arrest, he was hardly unaccustomed to being targeted by the police. During our walk around the Washington Park complex, he pointed to an area behind an apartment building. Cops, he said, used to drag residents there and beat them up. He recalled one officer who particularly “loved to whup Black kids.” Dozens of complaints were filed against him, Sawyer told me, but the officer was never disciplined. Sawyer said that since he was wrongfully arrested his anxiety in the presence of cops has spiked. “After that happened, I’m looking at the police like . . .” His voice trailed off.
A few months after Sawyer’s wrongful arrest, Carronne Jones was diagnosed as having diabetes, which she attributed to the stress of the experience. Why hadn’t the police conducted a more thorough investigation, she wanted to know, particularly after finding nothing in her sister’s house linking Sawyer to the crime? The police had also checked Jones’s car, at her urging, and come up empty.
In a 2021 law-review article, Laura Moy, the director of the Communications and Technology Law Clinic, at Georgetown, argued that the growing reliance on automated face recognition could lead to more wrongful convictions by displacing traditional investigative techniques, such as canvassing a neighborhood on foot and interviewing as many people as possible. These methods may require more time and resources, Moy noted, but they can expose false leads.
I eventually obtained the surveillance footage of the bus assault. Watching it, I immediately noticed how nimbly the assailant dashed away after stealing the driver’s phone. In Washington Park, Sawyer moved about gingerly, particularly on his right foot, which he strained to keep from pointing crookedly whenever he took a step. The condition resulted from a long-ago football injury that had never healed properly, he told me; for decades, he’d walked with a noticeably uneven gait. Why hadn’t this led any of the M.T.A. officers to wonder if the man moving rapidly in the surveillance video was really Sawyer? Seeing the facial-recognition results seems to have made them unable to see Sawyer.
The charges against Sawyer were dismissed on April 29, 2022—about a month after he was arrested. Neither he nor Jones received an apology from the police; nor were they alerted when another person was eventually arrested for the assault. The suspect, a man in his early thirties named Deon Ballard, is five feet eleven. The police visited the house of Ballard’s mother and showed her the Be on the Lookout bulletin. “Yeah, that’s my son,” she said. Later, Ballard’s picture was placed in an array of photographs and presented to LaDonna Wilson, the bus driver. Beneath Ballard’s photograph, she wrote, “Looks more like the man who assaulted me.” ♦
This post was originally published on this site be sure to check out more of their content.